Options
Palaniappan Ramu
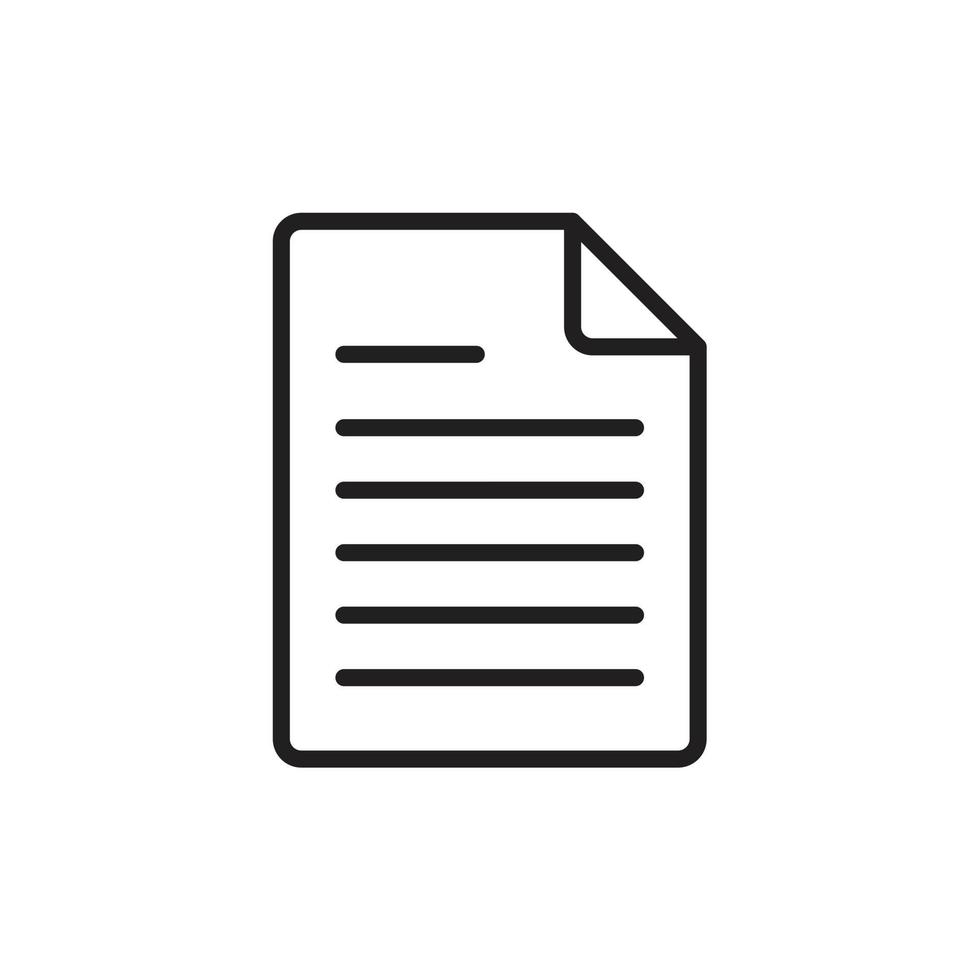
A dual surrogate driven L-moments based robust design with scarce samples in the presence of extremes
01-03-2022, Jayaraman, Deepan, Palaniappan Ramu, Suresh, Suhas Karkada, Ramanath, Vinay
Robust Design architectures permit identifying designs in the input space that minimize the mean as well as the spread of the response in the performance space, when the input variables are uncertain. Often, information about uncertainties is not readily available and are usually characterized by scarce samples that might contain extremes. Since extremes are part of the data, they cannot be excluded but including extremes alter the measures of spread such as standard deviation. Hence, it is imperative to develop a robust design architecture where the measure of spread estimations are less sensitive or insensitive to extremes. We propose using L-moments to estimate the measure of spread, the second L-moment (l2) and use it in the robust design formulation. We consider the cases of design variables which can be deterministic or random, and random variables. Hence, we use a dual surrogate framework where a design surrogate is built first. At each point in the DoE, scarce samples that might include extremes of the random variables are propagated through the design surrogate. Mean and measure of spread are computed by the L-moments approach at each point in the DoE, from the responses computed upon propagation, and used to build analysis surrogate which is used for identifying the robust design. The proposed approach is demonstrated on 2D Aspenberg function, 5D truss and 17D rotor disk design examples. The results reveal the superiority of the proposed approach over the conventional formulation.
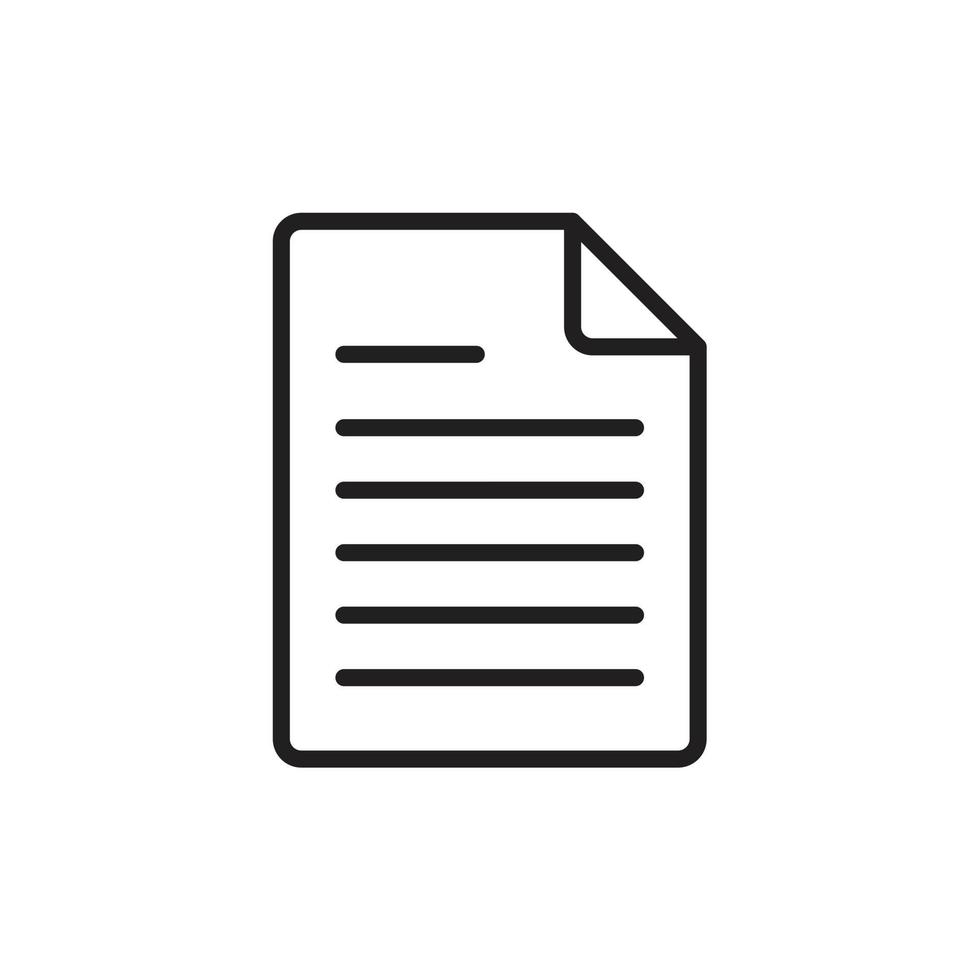
L-moments and Chebyshev inequality driven convex model for uncertainty quantification
01-07-2022, Jain, Naman, Palaniappan Ramu
In the design of real-world complex engineering systems and infrastructure, one typically deals with scarce data. When only scarce data are available, convexity approaches are widely used to construct the uncertainty model in terms of bounds. Convex models estimate the uncertainty bounds using different bounding geometries that seek minimum volume with the available points. Chebyshev inequality in conjunction with geometries such as convex hull allows accounting for unobserved future data points by inflating the initially constructed geometry. Since the Chebyshev inequality is independent of underlying distribution, the inflated convex geometry is often highly conservative. To address the conservativeness, we propose constructing a convex hull with a modified inflation coefficient that uses information on input dimension and spread of data. Since conventional metrics for spread of data such as kurtosis is volatile when sample is scarce, we use L-moments which are known to be robust to sample size. The initial convex hull built using samples from a distribution characterized by L-kurtosis, is inflated incrementally until allowable number of points corresponding to target probability are violated. The corresponding inflation coefficient is considered the modified coefficient. This exercise is repeated for different L-kurtosis and dimensions, and the modified inflation coefficient are obtained for different target probability as well to construct an empirical relationship between L-kurtosis, number of dimensions, and modified inflation coefficient. The proposed approach is demonstrated on three design examples, and the results reveal that the design from the proposed approach is less conservative compared to the classical approach while solving the probabilistic formulation deterministically with scarce samples. The performance also indicates that the proposed approach has good generalization and prediction capability.
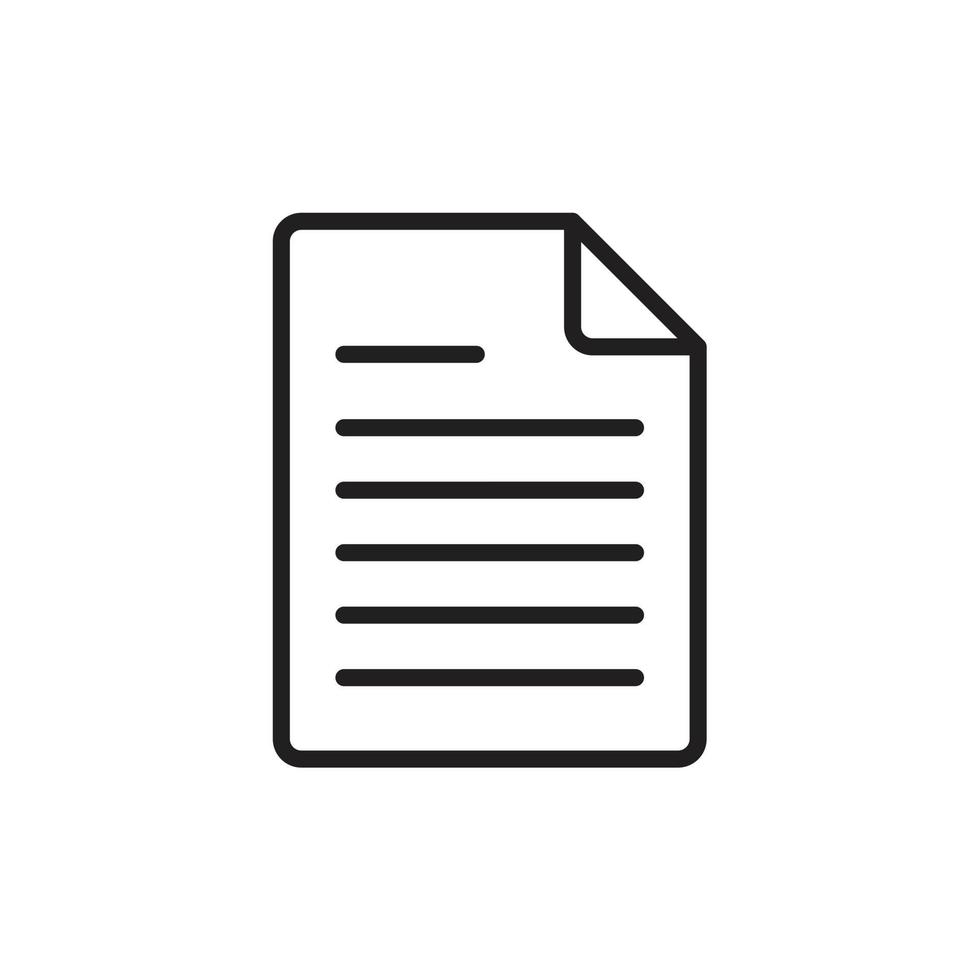
L-moments and Bayesian inference for probabilistic risk assessment with scarce samples that include extremes
01-07-2023, Jayaraman, Deepan, Palaniappan Ramu
In structural applications, uncertainties are unavoidable and play a significant role in risk assessment. Probabilistic risk assessment (PRA) is used to determine the level of risk associated with complex systems such as nuclear power plants, space missions, earthquakes, tornado and floods. The Bayesian inference is often regarded as an effective framework for analysing probabilistic risk and the prior probability and likelihood function are inferred from available data. The traditional approaches usually approximate likelihood functions using conventional moments (C-moments). If data are insufficient, approximations are inadequate, leading to inaccurate conclusions. Therefore in order to determine the amount of risk, it is desirable to develop a PRA framework that is distribution independent and less sensitive or insensitive to extremes in the scarce data. In this paper, L-moments are proposed to characterise or approximate likelihood functions in the Bayesian inference. L-moment ratio diagram is employed to select the appropriate distribution of conditional probabilities. The Bayesian approach is paired with L-moment approach to perform PRA and uncertainty quantification. The efficacy of the proposed approach is demonstrated on three engineering examples. In assessing risk from few samples in the presence of extremes, L-moments based Bayesian inference for PRA framework significantly outperformed its C-moment counterpart.
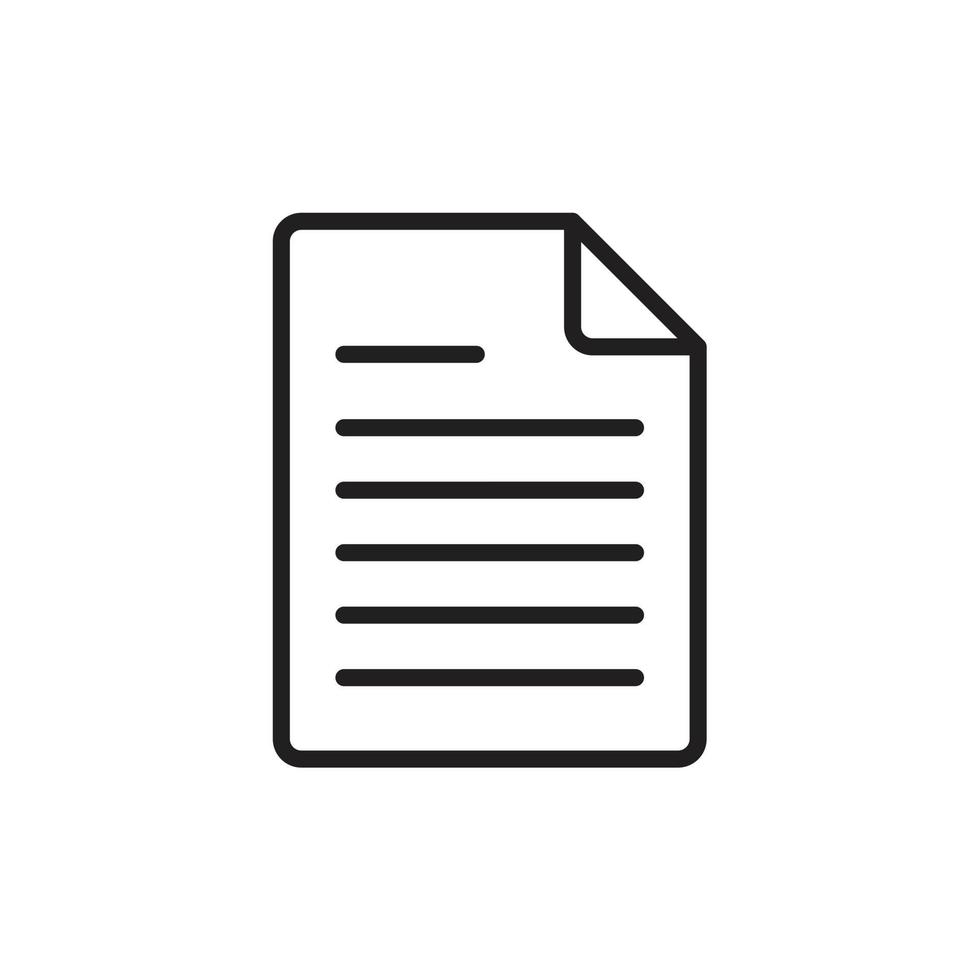
L-moments-based uncertainty quantification for scarce samples including extremes
01-08-2021, Jayaraman, Deepan, Palaniappan Ramu
Sampling-based uncertainty quantification demands large data. Hence, when the available sample is scarce, it is customary to assume a distribution and estimate its moments from scarce data, to characterize the uncertainties. Nonetheless, inaccurate assumption about the distribution leads to flawed decisions. In addition, extremes, if present in the scarce data, are prone to be classified as outliers and neglected which leads to wrong estimation of the moments. Therefore, it is desirable to develop a method that is (i) distribution independent or allows distribution identification with scarce samples and (ii) accounts for the extremes in data and yet be insensitive or less sensitive to moments estimation. We propose using L-moments to develop a distribution-independent, robust moment estimation approach to characterize the uncertainty and propagate it through the system model. L-moment ratio diagram that uses higher order L-moments is adopted to choose the appropriate distribution, for uncertainty quantification. This allows for better characterization of the output distribution and the probabilistic estimates obtained using L-moments are found to be less sensitive to the extremes in the data, compared to the results obtained from the conventional moments approach. The efficacy of the proposed approach is demonstrated on conventional distributions covering all types of tails and several engineering examples. Engineering examples include a sheet metal manufacturing process, 7 variable speed reducer, and probabilistic fatigue life estimation.